Mixed stochastic system design
Our goal is to establish the first-of-its-kind design methodology that (i) tailors the structural stochasticity and morphology simultaneously to achieve optimal performances and (ii) enables designing mixed stochasticity structural/microstructural systems. In our vision, this research will also lead to the automation of the nature/bio-inspired design process.
Stochastic modeling and computational design of microstructural materials
Our goal is to develop computational methods to enable microscopic image-based statistical characterization, stochastic reconstruction, numerical modeling, and uncertainty quantification of the heterogeneous microstructural materials. In addition, we are also interested in physics-informed statistical learning methods to discover the processing-microstructure-property relation for computational material design.
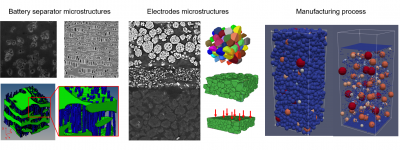
– Manufacturing process modeling to understand its impact on material microstructures and properties;
– Uncertainty quantification of the spatially-correlation random quantities in complex topological structures.
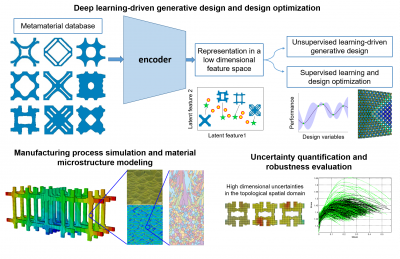
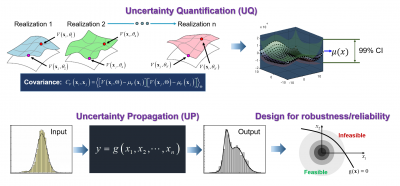