Mixed stochastic system design
Our goal is to establish the first-of-its-kind design methodology that (i) tailors the structural stochasticity and morphology simultaneously to achieve optimal performances and (ii) enables designing mixed stochasticity structural/microstructural systems. In our vision, this research will also lead to the automation of the nature/bio-inspired design process.
Ditigal and Cyber Manufacturing Systems: Resilience, AI-assisted Modeling, and Process Design
Stochastic modeling and computational design of microstructural materials
Our goal is to develop computational methods to enable microscopic image-based statistical characterization, stochastic reconstruction, numerical modeling, and uncertainty quantification of the heterogeneous microstructural materials. In addition, we are also interested in physics-informed statistical learning methods to discover the processing-microstructure-property relation for computational material design.
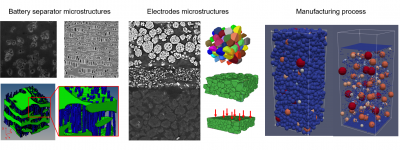
– Manufacturing process modeling to understand its impact on material microstructures and properties;
– Uncertainty quantification of the spatially-correlation random quantities in complex topological structures.
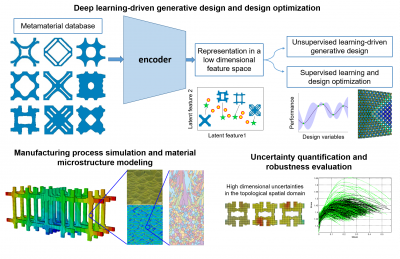
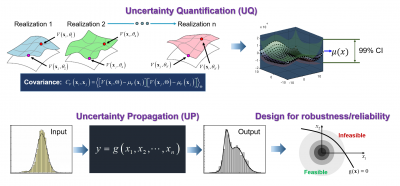
- NSF – CAREER: Bridging the Gap between Deterministic and Stochastic Structures for Mixed Stochasticity System Design (ongoing)
- NSF – Collaborative Research: Understanding the deformation-morphology-conductivity relationship in multistage forming-based manufacturing of conformal electronics (ongoing)
- NSF – ENG-EAM: Collaborative Research: Embedding Stoppage-free Resilience to Stealthy Cyberphysical Attacks in Digital Manufacturing Systems (ongoing)
- DOE – Control of Aerospace Manufacturing Variability Using Physics-Informed Artificial Intelligence (ongoing)
- NIUVT – Modeling, Experiments and Prototype Development of Near Theoretical Capacity Lithium Ion Batteries for Unmanned Underwater Vehicles (ongoing)
- GVSC via DREAM Center – Computational Design of Stochastically Graded Structures for Stress Wave Manipulation (ongoing)
- GVSC via DREAM Center – Modeling and Design of fluid infilled complex structures for mechanical performances (ongoing)
- NIUVT – Design, Additive Manufacturing, and Testing of Phononic Bandgap Metamaterials for Controlling Mechanical Waves (ongoing)
-
Ford Motor Company – Computational design of lattice structures for vehicle impact performance with structural uncertainties induced by AM process (completed)
-
DOED – UConn-GAANN: EnCoDiT: Engineering Cognitive Digital Twin Technologies For Predictive Design And Manufacturing (completed)
- SHAP3D – Deep Generative Design of Cellular Metamaterials for AM (completed)
- UConn CARIC internal grant – Virtual Investigation of Structures Using an Intelligent and Optimized Digital Network (completed)
- UConn REP internal grant – Elastic Metamaterials as a Generic Haptic Interface for Virtual and Augmented Reality (completed)